'Natural' Language Processing. Is it though?
Are we overestimating the machines or misprizing mimesis? What if we're ill-equipped to tell! At least, let’s give the children in your life due credit, shall we?
Should people in day jobs that require regular analytical writing and research worry if AI will take their job or at least hurt the pay they might expect in the position they aspire to in five years? The standard approach to reporting on natural language algorithms can leave you uncertain. The question needs more careful thought than it gets.
You have likely heard of the most vaunted of AI players in this field. GPT-3 is the largest of what is called a large language model. Let’s call it GPT for here. Its primary skill is to produce descriptive or analytical prose on demand. You give it a few words or sentences. It expands with many more, on that subject, in that style.
The earliest articles on GPT came in a simple two-part structure. The author would tell you the prompt they fed it and paste a copy of the algorithm’s response. The author and you would then together gasp at how lucid it read. In the period when the software was open for anyone to tinker with, many did. The odds that testers would stumble upon a gaping flaw were high back then. The structure of articles in the second phase got a bit more nuanced. Of course you got the prompts and response, but now, writers included cautionary tales from some of the testers. Farhad Manjoo spoke to Janelle Shane. She asked GPT to expand on some facts about whales. It supplied lies. Maybe it was just trying to fit in with 2020.
In April, the Times returned to check on the progress with GPT, and this was a longform article. There was plenty of space for a range of outlooks. Parts suggested that GPT might soon shrink workspaces as different as lawyers and software engineers (though both work with ‘code’). But the piece was also seasoned generously with criticism from experts in the field: “blind mimicry”, ‘‘stochastic parrots’’, “pastiche generation”. As with the whales, Steven Johnson also found that GPT merrily played along with his invention of a nonexistent Belgian chemist, even offering up a date and place of birth. Then he spent acreage on an elaborate history of OpenAI, the consortium behind GPT and the evolution of their vision. I learned a great deal. But the considerable length by that point didn’t mean you were left wanting for GPT responses to prompts of more sophisticated, updated kinds.
The example Johnson chose was to compare “the music of Brian Eno to a dolphin”. I always find the accomplished human writer’s response to AI responses illuminating. Allowing that parts of GPT’s responses are awkward, he brushes it away, as a doting uncle might, because the “prompt itself is nonsensical”. Then the obligatory human comparison: “If you gave 100 high school students the same prompt, I doubt you would get more than a handful of papers that exceeded GPT’s attempt.” I have actually taught high schoolers as a volunteer briefly in Netherlands and India. Most teenagers I know would just say that the task is nonsensical, right after being told what a brianeno is. If forced to put it on a page, that would be their first line. Remember, it wasn’t ‘compare the song of dolphins to’, it was ‘compare dolphins to’. Not to impugn the skill of American teenagers, of course. As the promotion for this newsletter promises, lets look back to the Times. Here’s a collection of brief essays written by high school students1 that had me reassess my own writing ability! Can you imagine one of those kids concluding that “both dolphins and Eno’s music often defy categorization, and can be difficult to define”. Tut-tut GPT…! No, dolphins are much easier to categorize. It may have taken us centuries to get their slot right, and we still have some work to do, but you were definitely overstating it there!
But we are used this by now, the comparison to kids. Not arthropods or cephalopods, directly human kids! (Cyclopods?) MIT Technology Review’s Karen Hao is a veteran tech reporter I have learned a lot from. (If you’re into AI, you want to follow her on all platforms.) Her verdict on some of GPT’s 2020 attempts at creating images in response to text prompts was that were the equivalent of “a child’s stick figures” to represent people. Hao is not some AI votary. Her phrase for describing the text-only output of GPT at the top of that same article was “parlor trick”. And if you saw the output images she is referring to—"woman attempting to ski”—you too might think she’s on point. That’s because humans are programmed to see more than there is to see when we are primed by words that tell us what to look for. Jesus on toast, man in the moon… you get the IDEA. We tend to not mind that the woman appears to have three legs! It’s delectably meta. People give a machine text prompts > machine makes images > but people’s brains perceive by predicting2 > so people are not the best judges for the test they set! (Actually better than meta, it’s recursion, but I digress. Ask me some day over tea.)
The comparisons to young humans give short shrift to the splendor of a human child’s brain and leave readers with a false impression of both, the progress we can soon expect in AI and appropriate benchmarks to assess a child’s progress. What a child is doing in a stick figure is deducing a working model of a part of their world—a conceptual model transposed onto paper for communicating with others. The child has more than met the burden of proving that they understand the mechanics of a human body—limbs, torso, head and their relative positions given their functional roles. The only comparison to the GPT images is in demonstrating that the machine has not managed to deduce anything close to a conceptual model. How could it, when it was programmed only to classify which visual elements statistically correspond to what words. It is a mathematical model3 with extremely constrained use cases.
The more urgent question I had was, when journalists are given access for this sort of testing, to what extent are their prompts prompted by the AI’s engineer parents? You know, the way when your parents had visitors, they didn’t let the visitors pick any poem at random for you to recite. They know the one you can. Sure, Johnson chose the actual prompt. But was he helpfully nudged, “Try asking it to compare two things, any two random things, however weird”? Pick a card… any card. Parlor trick, except with the tech reporter as an unwitting shill [*]. The syntax of the sentences in the response sort of rubs me that way.
In technical terms, it’s what my econometrics professor at grad school called the ‘rabbit in the hat trick’. You write a complicated economics model in a way that it has a rabbit concealed in it, sometimes oblivious to it. You run the model on a dataset of exactly 49 countries and lo—the model predicts the rabbit! Engineers understand that metaphor all too well. It is much easier to design a mathematical model that responds in predictable ways for a selected set of inputs than it is to design a working solution to a real-world problem. My stoichiometry professor at college too warned us that we’d fail the undergrad project if he smelled a rabbit, without niceties like metaphors.
Comparisons to children somewhere along the way are a problem at one level. It is also customary to end these articles with an implicit comparison to trained adult professionals, that is, you. Usually, they hint at the near future. Not this time. After listing GPT’s several deficiencies, then pivoting to significant social issues, the Times firmly declares fait accompli!
GPT-3 and its peers have made one astonishing thing clear: The machines have acquired language.
That sounds at once final and unsubstantiated. As is this newsletter’s wont, let’s then try and steelman this whole machines-can-now-speak thing. For the first thing, my ears pricked at the mention of ‘‘multimodal neurons’’:
…it became activated not just by images of spiders but also by illustrated Spider-Men from comic books and… by images of the word ‘‘spider’’ spelled out.
Where have I heard that before? Oh yes, I have been following work at the Gallant Lab at Berkeley. Recent updates in their work suggest that in the human brain, images associated with a concept are consistently encoded near corresponding words. Note that it’s ‘near’. It’s not the same neuron, which is a level of detail I gather we can’t zoom into yet anyway. I don’t understand enough to tell you what that difference signifies. What I can remind you is that humans learn things in a profoundly different way to algorithms. Toddlers do not learn to distinguish cats from dogs or associate spider the arachnid with ‘spider’ the English word for them by zapping through millions of images and pages. We first teach them very complex Wittgensteinian grammar. Or rather, they acquire it. Only then we tell them ‘this <is> a cat’
(usually in the presence of a cat) and ‘spider <is> spelt S-P-I-D-E-R’
.
The angle brackets are to somehow mark the logic rule embedded in the word ‘is’. That’s what Panini’s rough notes must have looked like. Because a toddler has a firm grasp on ‘is’, she only needs to see a cat twice before the furry animal merits that permanent space in her brain for the visual and the matching word. Of course, everyone knows this, even if we don’t associate ‘simple’ sentences kids utter with the complex logical propositions that they are. But inside articles about AI algorithms you’d not know that everyone knows it.
There is a lot more to say on the subject of learning, but perhaps it’s best reserved for later issues. For now, the above is only to underscore that the only way babies learn anything is by being around other humans. Why anyone thinks machines can ‘learn’ something all on their own4 in a cold dark basement and acquire ‘intelligence’ escapes me.
Then again, for all our sophistication, why is that credible mimicry even possible? In another section Johnson says:
…Perhaps the game of predict-the-next-word is what children unconsciously play when they are acquiring language themselves
That again gave me great pause. I thought with a shudder, young second-language speakers indeed often keep notes of new phrases they hear, as a memory aid. Next time they are in a conversation that turns around a similar context, they can toss the phrase in and sound smarter than they could otherwise hope to. Sounding familiar yet, dear stochastic parrot? Even as an adult, I have kept such written notes in French and Spanish and mental ones for Bangla, Dutch, Hungarian, Marathi, Punjabi and Swedish. Perhaps native speakers keep a version of these mental notes too? But surely, not in English, I thought. We frown upon speakers and writers who ‘promptly’ supply a well-worn cliché after an almost audible click when the opportunity presents itself.
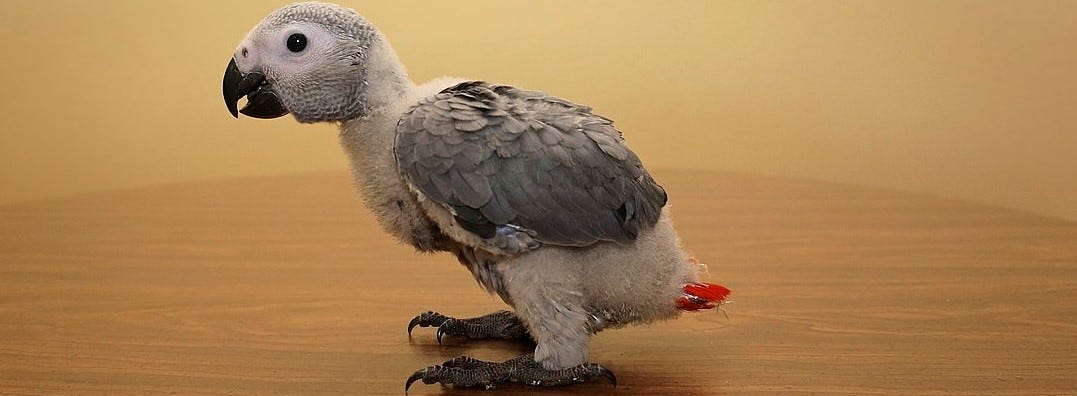
Time to check with an expert. Yohei Igarashi, a professor of English, anticipated precisely this question just last September in Aeon. He in turn points to someone who wrote of this in 1971! Surprise doesn’t come close to describing my reaction to Walter J. Ong’s thesis. In brief, the earliest literary traditions were oral (something I should know only too well, having being taught as a child to recite numerous couplets in a language no one I knew actually spoke). Oral literature relied on repetitive structures, and even stock phrases, as a mnemonic aid. That was once the most efficient way to pass knowledge, which is what makes us ‘Sapiens’ in the first place. It was technology—the printing press5—that in fact made way for a shift in stylistic preferences away from ‘formulaic composition’.
You see the paradox too, right? A premium on originality is what brings you innovation in the first place! Past technological progress liberated our creativity. Our latest efforts are making us regurgitate rehashes of past marketing copy all over webpages and pay top dollar for it!
And yet, Igarashi notes:
the way computers can now write resembles how humans first spoke…Even so, one of the stranger effects of contemporary language models is that they reveal to us that our plain style is itself full of highly probable phrases. …our post-commonplace writing is actually full of commonplaces.
He goes on to suggest all writing is a virtual palimpsest, except that we, even humans, don’t just write over the old words, we trace over many of them. The article put me in mind of cable news anchors and late-night comedians who indeed seem to be speaking by variously filling blanks in tired snowclone after another, day after day. (You might even now be thinking of that colleague.) And writing? The tropes reused in coverage of AI algorithms certainly don’t help argue the case against. In fact, didn’t I start this post with just that suggestion! Sometimes, it is possible to do too good a job at steelmanning someone’s case. 😬 If the Times had cited Walter J. Ong, I would have struggled hard against my instinctive resistance to hype around AI.
The essay concludes in a cheerless place: perhaps “we collectively long for the banal” and the ideal “ratio between the familiar and the fresh” is “elusive”.
I still don’t like the sweep of that. Maybe some or much writing is pastiche in one way or another. Yes, every human is the sum of countless others. But that is not the same thing as when we say, for instance, that all science stands on the shoulders of giants. Predicting words governed by syntax and norms of usage or style is at least one quantum order below the tracing of a logical or organic progression in ideas.
When Adam Tooze propounds a thesis to explain a socioeconomic phenomenon, sure he may arrive at a formulation that superficially matches a thought first recorded in Mandarin in a forgotten age or still repeated in oral Maori traditions. But he may arrive at it independently, after reading exhaustively on his chosen context. When N.K. Jemisin sits down to sketch an imagined world for a new novel, sure she relies on general principles of geology and its influence on evolution of species, but the specifics are all unique and novel. You might argue both their output is influenced by a blend of all work they have consumed in the past in a direct if non-deterministic way. But I like to hope some humans have an exceptional, inimitable talent for originality. We’d do well to nurture it rather than snuff it out of the marketplace.
No, I’d rather end around a more optimistic proposition that Igarashi skirts by:
…older technologies of writing… freed up the human mind from the burden of information storage so that we could be more creative. Likewise, today’s text technologies, which can generate serviceable writing, need not kill off the idea of human originality so much as reinvigorate it – a new Romanticism.
The question is, are most engineers and entrepreneurs designing algorithms to assist humans [*] or to replace them? As an engineer I can tell you those are very different pursuits. Think of a huge industrial saw at an IKEA plant versus one of those German handheld DIY drills that you could use at home to build your next furnishing magnum opus, perhaps to equal your child’s imagination. As an economist, I can tell you they lead to diametrically different answers to that question in the subheading above.
Here’s hoping that AI labs, many of whom already engage with neuroscience deeply, realize that the two approaches diverge at an early fork. One road is for Pollyannas chasing mirages, sucking in investment and resources at immense opportunity costs. The alternative is to build AI coworkers for us all. Sure, you cannot expect ever higher incomes and promotions based on cumulative ‘work experience’ alone. In many industries, we have been in that world for some time now, with or without AI. Only now, upskilling will mean learning to work with your new machine coworker. Crucially, you would not lose your job to a machine, because the machine was designed ground-up to augment you. The employer aspires to better than the machine can do on its own. Because only together, humans and machines, we avoid the specter of that endless monotonous future where everything ‘new’ is a repackaging of the past.
Post Date
17-11-2022: A 2021 PNAS paper I just came across. It reports some evidence that the brain might share the predictive verbal processing of some NLP models. Here’s an explainer for non-academics at the MIT website. They only compared the visible activity of ‘nodes’ though, so not direct evidence on the underlying processes.
The reasons are adjacent to some of the research I referred to in the May 20 issue on religion. It will come up in some detail in future issues. (Not to leave you in a muddle, but hope you’re not linking “human brains lie” and “brains constantly predict” from that post to claims about lies and predictions of algorithms here. Even though AI researchers speak of writing neural networks inspired by brains, the two sets are entirely unrelated phenomena. The May 20 post was about visual perception, this one is about language composition structure.)
And not a very good one, because in any decent mathematical model, humans can trace what drives what.
Machines are beginning to learn from one another too, but of course that means they remain confined to mathematical models.
This too now rings bells. After all, both our words cliché and stereotype come directly from parts of the early printing press!